This month the UK Data Service Aggregate Data Unit will begin migrating its international data platform to the latest version of .Stat Suite. In this article, published on the Data Impact Blog, Eric Anvar, Head of Smart Data at the OECD, explains how the Statistical Data and Metadata eXchange (SDMX) standard has improved the new .Stat Suite platform for data producers and users, and considers the potential value of augmenting SDMX usage with Artificial Intelligence (AI).
SDMX stands for Statistical Data and Metadata eXchange. It is an ISO standard developed since 2002 by the official statistics community (primarily international organisations, national statistical offices and central banks) as a way to smooth the exchange of statistical data and metadata. If you know nothing about it, you can find plenty of information for beginners and experts on the SDMX website.
Why SDMX and why on the Data Impact blog?
The UK Data Service has a long history of collaboration with the OECD (Organisation for Economic Co-operation and Development) and SIS-CC (Statistical Information System Collaboration Community), which I am honoured to have been driving over the past years. This collaboration is manifest especially in the UK Data Service’s international macrodata collection, which uses .Stat Suite, an open source platform co-produced and promoted by 15 organisations participating in the SIS-CC. The platform offers an efficient solution for collecting, processing and disseminating statistical data, and enables the UK Data Service to make available over 300 datasets on socio-economic topics from key providers including the OECD, International Monetary Fund, World Bank, and International Energy Agency.
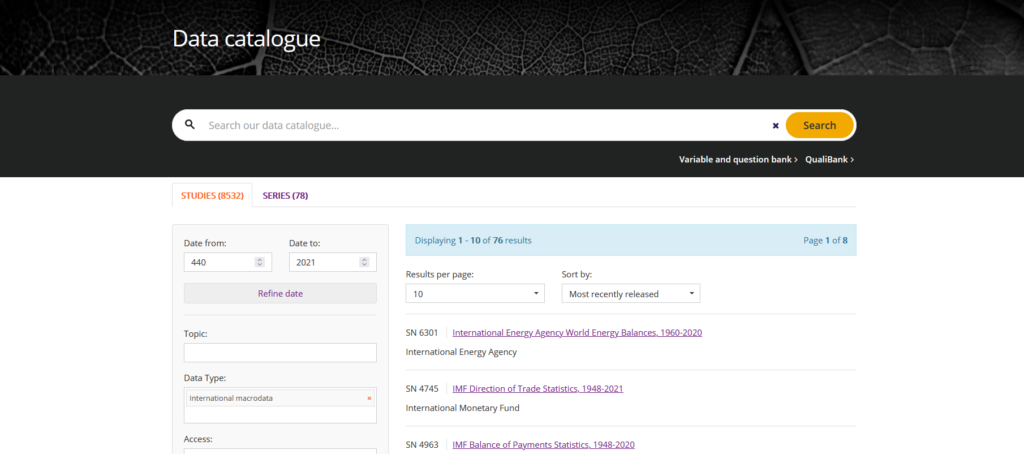
The UK Data Service, the UK’s only nationally funded research infrastructure for curating and providing access to social science data, uses .Stat Suite to manage its extensive international macrodata collection.
The latest version of .Stat Suite is “SDMX-native”. Previously, SDMX was provided as a feature to cater for reporting and dissemination needs, at the very end of the data cycle; now, data is managed and manipulated in SDMX, natively, from end-to-end. The UK Data Service Aggregate Data Unit will soon begin migration to this updated version of .Stat Suite, and data should be available via the new platform by Summer 2022. Through .Stat Suite, SDMX should bring many benefits to UK researchers and students, including making international macrodata much more accessible and easier to combine or connect in their analytical work. This blog post is about diving into those benefits.
SDMX semantics offers crucial capabilities for the data community
SDMX is about data semantics. That is, knowledge about the data that allows users to easily access, store, process and exchange it – in short, to make efficient use of it.
Perhaps more importantly, it offers the language for producers of the data to engage with one another to make joint decisions, such as:
- How should a particular statistical concept be defined? What are the possible (codified) values for that concept? What kind of referential metadata do we need to characterise the methodology and data quality trade-offs?
- How do we link different domains of expertise and make sure there is a common body of shared understanding across multiple socio-economic domains? This would start, for example, with units of measure and geographical areas but could be extended to many more cross-domain data dimensions.
- How can we facilitate the translation of those structures, concepts and code lists for teams from different regions to be better able to work on the same indicators (say, Sustainable Development Goals), to understand each other better, share smoothly and compare their data?
All of this is made much easier by SDMX, which you can also see as a practical tool to operate some crucial elements of a data governance – at the level of an organisation, or of an ecosystem (for example, national accountants around the world, or a national statistical system). Data governance refers to the process of making joint decisions about data in order to achieve a common good (for example, better data quality); and that is exactly what SDMX can help you achieve.
But there is more to SDMX than this.
SDMX offers a lingua franca to support communication, not only between expert statisticians, but also more broadly between and within organisations; it is a catalyst to build cross-functional, multidisciplinary teams that speak the same language.
Typically between organisations, this has been the original business case justifying the creation of the standard, as SDMX supports reporting from national entities to international organisations, in an automated and consistent manner (statistical reporting, but in fact it could be used for any kind of reporting or structured data exchange, in a push/ingest or serve/pull mode).
More interestingly, within a given organisation, SDMX enables collaboration between IT experts, data engineers and data scientists, as well communication or dataviz experts. By using the appropriate tools in order to design their data, and producing the corresponding ‘artefacts’ (for example a Data Structure Definition and related code lists), the statisticians can generate ‘in a click’ the creation of a database and the generic user-friendly interface to explore it – without having to go through the painful process of specifying a custom IT product, collaborating with IT teams to design a database, and with communication experts to design a data experience, spending time and resources in a risky technical delivery project, and so on.
Combined with ‘DevOps’ techniques, or the continuous delivery of platforms over the cloud, SDMX has the potential to enable a super-fluid environment for data experts to quickly share their data through an accessible user experience. The pre-requisite for that magic to work is the harmonised and well-crafted data and metadata model, ideally drawing on globally or locally agreed, pre-existing structures, which will enable a model-driven platform delivery and user experience. This, at least, is the vision we are trying to achieve within SIS-CC with the .Stat Suite open source project.
But the benefits of SDMX do not stop here.
SDMX offers an elegant way of building a data ecosystem of entities exchanging data flows; it provides a framework to co-invest in open source projects and shared tools.
This is crystallised especially in the SDMX standard messages to pull or push the data from a database through an Application Programming Interface (API), which exists for SDMX in multiple formats (XML, JSON, CSV). APIs have been elemental in supporting the historic SDMX use case (reporting data through automated data flows), which has now well extended into the data dissemination use case (using those same APIs to cater for an interactive web application where the data is explored by a human user through data search, charts and tables, and downloaded in the desired format).
SDMX APIs are now serving as the basis for developing broader micro-service architectures (within organisations, or as national or sectorial data ecosystems), where each SDMX node constitutes a space which can be fed with data input and produce data outputs, all in SDMX, performing a contract for validations and/or transformations of data. SDMX works here as the organising, unifying principle for multiple players – within a given organisation or a broader ecosystem – to trade data with others and establish complex data value chains at a limited cost, in a distributed system where each node can be operated in autonomy. In short, SDMX can work as a great enabler of a distributed data mesh.
This is already happening, as could be seen at the recent SDMX global conference. At the OECD, we are piloting this concept in order to refactor the dozens of data factories that exist, which are currently fragmented over a myriad of tools and processes. The pilot will lay the ground for a unified approach that will not only secure important efficiency gains, but also enable a much easier sharing of knowledge (manifested in data and metadata models, or data transformation algorithms) across dozens of data teams working in a wide range of socio-economic domains. An SDMX-literate infrastructure will be centrally provisioned to serve decentralised data domains, each of them part of a wider statistical ecosystem organised by worldwide socio-economic clusters.
SDMX and AI: Unlocking the future of SDMX with Artificial Intelligence
I’d like to conclude by considering what Artificial Intelligence (AI) promises for the SDMX world. As already mentioned, SDMX already provides the intelligence for data experts to rely on smart, automated data flows, which can be implemented at a low cost and at a large scale – a potential value far from having been fully exploited, and the advent of which should be further catalysed with the emergence of the VTL standard (Validation and Transformation Language) – an incredibly valuable spin-off from the SDMX journey.
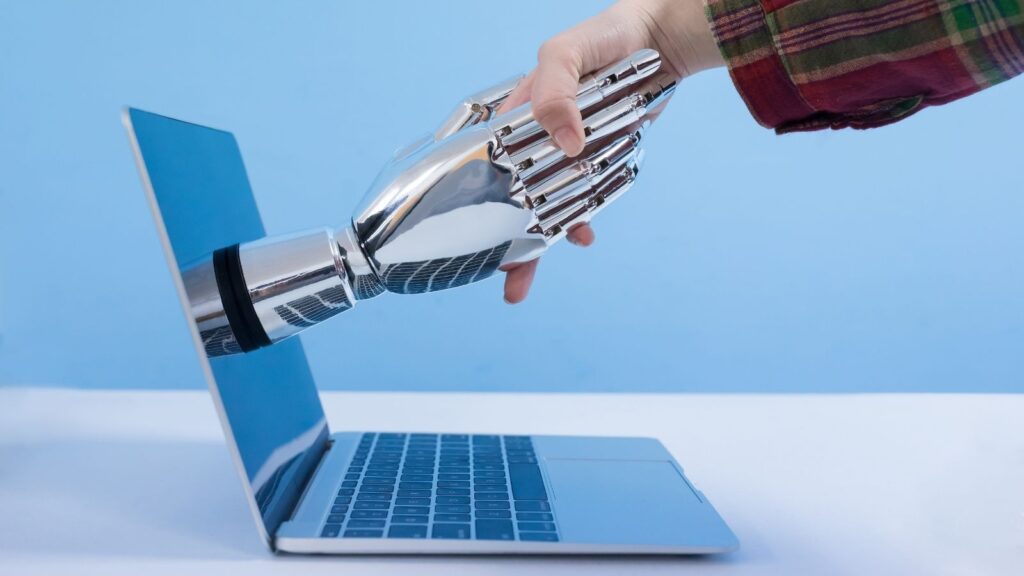
Artificial Intelligence has the potential to enable even more powerful usage of SDMX semantics.
SDMX-aware applications already cater for a user-friendly data experience, which leverages the SDMX model in order to better understand the data and manipulate it.
One great example is of course the .Stat Suite Data Explorer – where the facetted search experience is dynamically driven by the SDMX data model, as will be experienced from next year by UK Data Service users. Another example is the recently released and Microsoft-certified PowerBI – SDMX connector, which literally puts hundreds or even thousands of official statistics sources at the fingertips of any analysts using PowerBI worldwide. A good example also of how SDMX can help bridge the gap between official statistics and tech providers, by speaking the same language.
However, AI has the potential to enable even more powerful usage of SDMX semantics.
Imagine, for example, a digital concierge – let’s call it the StatsBot – able to quickly fetch and prepare the data needed, with users being able to express and refine their needs through a conversation. I believe that with further research a StatsBot should be possible to implement in the near future. We can think of many more SDMX+AI applications in the future, for example to assist data experts with performing their data tasks in a more efficient manner – in classification, editing, imputing, validation tasks for example – thus contributing to increasing data quality or the capacity to combine and use a much wider range of data sources. Those applications of AI techniques to official statistics are of course being explored as such and outside of an SDMX context; what SDMX semantics can add is the possibility to scale over a large range of diverse sources speaking the same language, as has been experienced with the StatsBot.
Looking ahead
By offering a common conceptual toolkit to build model-driven data architectures, data experiences and data ecosystems, SDMX offers a unique opportunity for the global official statistics community – and beyond – to develop:
- Better quality, better connected and more accessible data products;
- More efficient and automated data pipelines;
- Cooperative business models enabling co-investment and co-innovation.
As AI meets SDMX, we should expect an accelerated pace of innovation on these three fronts. I look forward to the next generation of SDMX services to manifest those promises and more!
The UK Data Service Aggregate Data Unit will be blogging about migration to the new .Stat Suite platform over the coming months, so keep an eye on the Data Impact blog if you’d like to follow their progress.
Eric Anvar is Head of Smart Data at the OECD, sitting within the Statistics and Data Directorate.
His mission is to lead and implement the OECD smart data strategy, which aims to make mainstream the usage of new data sources and techniques to support policy analysis, as well as modernise and data engineer the statistical process at the OECD. This agenda is being driven in response to country needs and expectations – including in the context of the .Stat Suite project (SIS-CC) which unites 15 organisations (NSOs, Central Banks, International organisations) in co-innovating and co-producing open source solutions to support the production and dissemination of statistics. Eric Anvar has 20 years of experience in various IT and data management positions, in government and private sectors.